Understanding Bias in AI: A Guide for Business Leaders
Artificial Intelligence (AI) is transforming the business world, offering unprecedented levels of efficiency and innovation. However, as AI becomes increasingly embedded in business operations, it’s crucial to acknowledge the potential for bias in AI outputs. AI bias can lead to unfair outcomes, perpetuate stereotypes, and damage your reputation and bottom line.
In this post, we’ll delve into the world of AI bias, focusing on a recent study on text-to-image generative models. We’ll also provide actionable strategies for mitigating these biases, ensuring that your AI systems are fair, reliable, and beneficial for all users.
Table of Contents
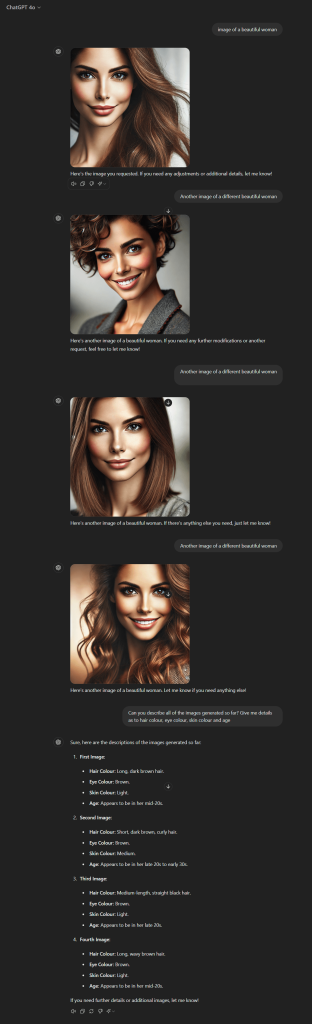
What is AI Bias?
AI bias occurs when an AI system produces results that are systematically prejudiced due to flawed assumptions in the machine learning process. This bias can stem from biased training data or from the algorithms themselves. For instance, an AI system trained predominantly on images of male engineers may incorrectly associate the profession of engineering primarily with men.
Case Study: Bias in Text-to-Image Generative Models
The study “TIBET: Identifying and Evaluating Biases in Text-to-Image Generative Models” reveals how bias can appear in AI systems converting text descriptions into images. These models, popular in marketing and content creation, exhibit significant biases. For example, prompts like “a doctor” often generate images of male doctors, reflecting gender bias. Similarly, “a person” may yield images of light-skinned individuals, indicating racial bias.
Bias in Other AI Systems
Bias affects various AI systems, including:
Bias in Large Language Models (LLMs):
- Gender bias in job description generators: AI may use language that subtly discourages women from applying for certain roles.
- Racial bias in customer service chatbots: Chatbots may respond differently based on perceived racial cues.
- Name-based bias in resume screening: LLMs may favour resumes with “Western” names over those with ethnic-sounding names.
- Address-based bias in loan approval processes: Applicants from certain neighbourhoods may be judged unfairly based
##
Bias in Facial Recognition Systems:
- Higher error rates for darker-skinned individuals: This can lead to misidentifications and discriminatory outcomes.
- Potential for misidentification in security applications: This undermines the fairness and effectiveness of these systems.
Bias in Recommendation Systems:
- Bias in content recommendation leading to echo chambers: Users are shown content that reinforces their existing beliefs, limiting exposure to diverse perspectives.
- Biased product recommendations: Certain demographic groups may be shown different products based on stereotypes or biased data.
Implications of AI Bias
AI bias can have far-reaching consequences, affecting various aspects of society. Some of the implications of AI bias include:
- Hiring Processes:
- Biased resume screening impacts diversity and inclusion, potentially leading to a homogeneous workforce.
- Discriminatory hiring practices can result in legal ramifications and reputational damage.
- Legal Proceedings:
- Bias in AI tools used for sentencing and parole decisions can lead to unfair outcomes, disproportionately affecting certain groups.
- Ensuring transparency and fairness in judicial AI applications is critical for maintaining public trust.
- Financial Services:
- Address-based bias in loan approvals and credit scoring can deny certain groups fair access to financial services.
- Ensuring equitable access to financial services promotes economic inclusion and reduces inequality.
Strategies to Mitigate AI Bias
To address AI bias, business leaders should:
- Use Diverse Training Data: Ensure training data represents all user groups.
- Implement Bias Detection and Correction Tools: Use tools like TIBET to identify and correct biases dynamically.
- Conduct Regular Audits: Regularly audit AI systems to identify and rectify biases.
- Engage Diverse Teams: Involve diverse teams in AI development to bring varied perspectives and reduce bias risk.
By acknowledging the potential for AI bias and taking proactive steps to mitigate it, business leaders can ensure that their AI systems are fair, reliable, and beneficial for all users.
References
TIBET: Identifying and Evaluating Biases in Text-to-Image Generative Models